
Community Spotlight: How I built llama2.🔥 by Aydyn Tairov
Mojo SDK was released in September 2023. As someone who relies on the simplicity of Python and also cares about high performance delivered by languages like C, I was excited to try out Mojo. I felt the same joy and thrill I had experienced when I first discovered programming and ran “Hello World” in QBasic and Turbo Pascal.
.png)
Using Mojo🔥 with Python🐍
Mojo allows you to access the entire Python ecosystem, but environments can vary depending on how Python was installed. It's worth taking some time to understand exactly how modules and packages work in Python, as there are a few complications to be aware of. If you've had trouble calling into Python code before, this will help you get started.
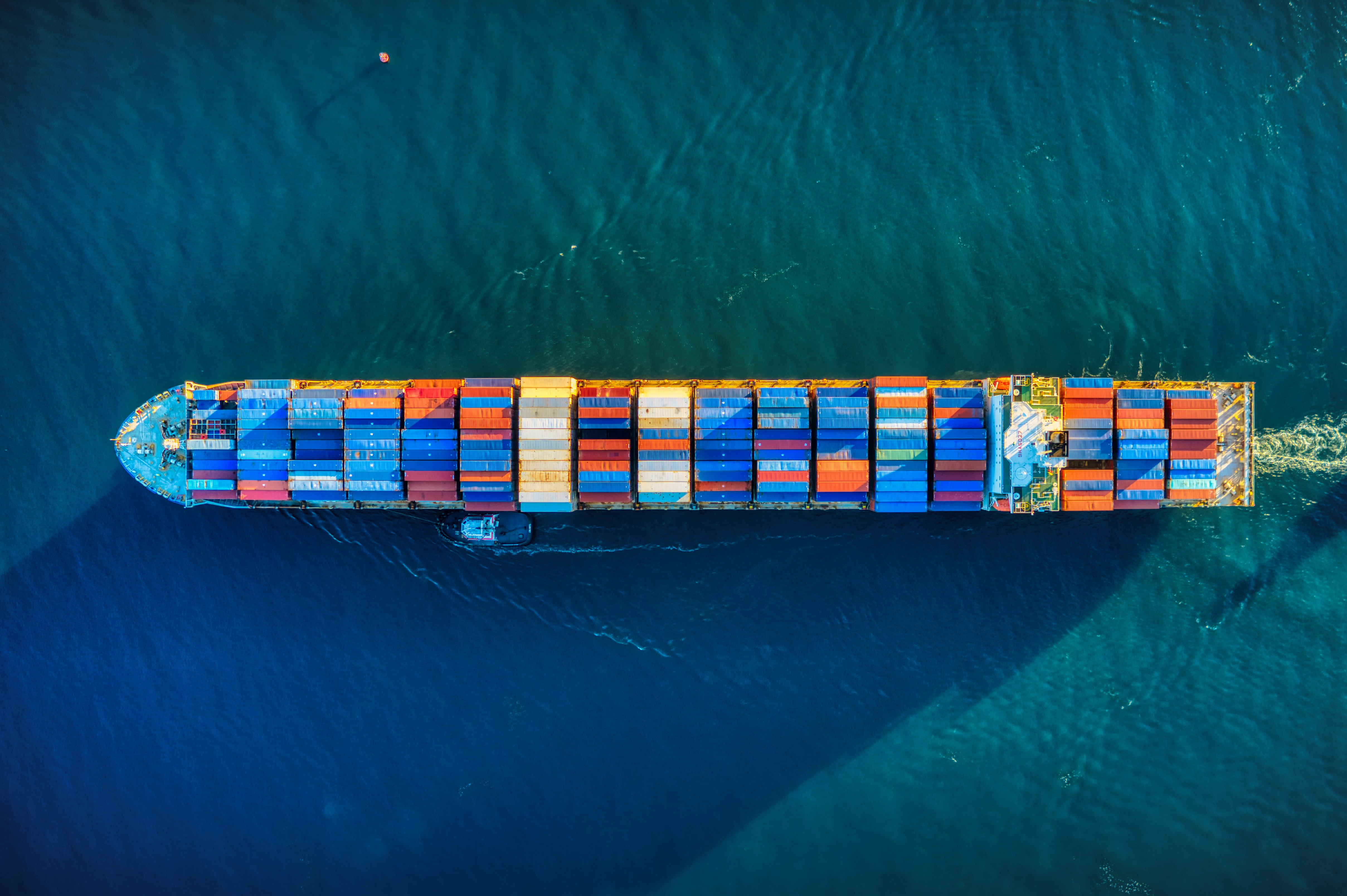
How to setup a Mojo🔥 development environment with Docker containers
How do you guarantee that your software is portable, runs reliably, and scales easily in production environments? The short answer is: Use containers. Container technologies like Docker and Kubernetes are popular tools for building and deploying software applications, but until recently they were considered exotic infrastructure for IT/Ops experts.
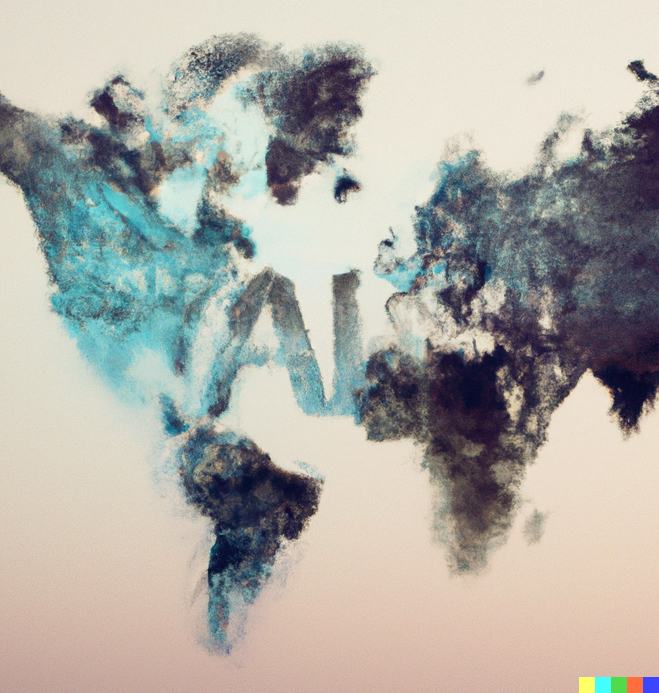
AI Regulation: step with care, and great tact
AI systems take an incredible amount of time to build and get right - I know because I have helped scale some of the largest AI systems in the world, which have directly and indirectly impacted billions of people. If I step back and reflect briefly - we were promised mass production self-driving cars 10+ years ago, and yet we still barely have any autonomous vehicles on the road today.
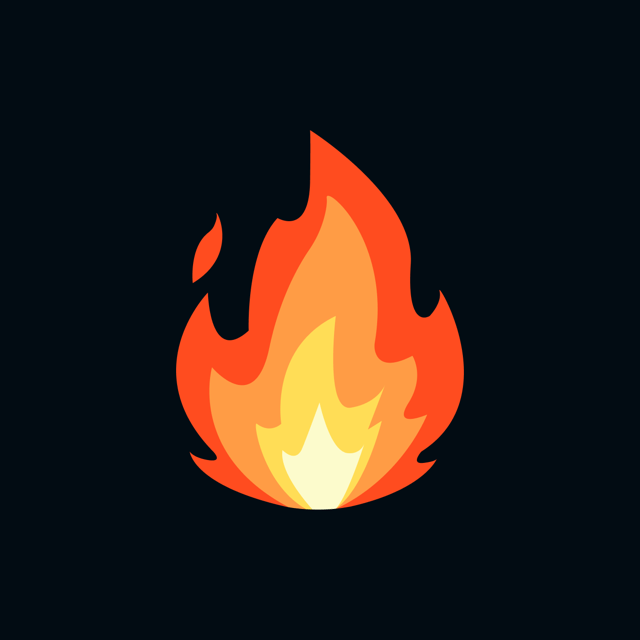
Mojo🔥 - It’s finally here!
Since our launch of the Mojo programming language on May 2nd, more than 120K+ developers have signed up to use the Mojo Playground and 19K+ developers actively discuss Mojo on Discord and GitHub. Today, we’re excited to announce the next big step in Mojo’s evolution: Mojo is now available for local download – beginning with Linux systems, and adding Mac and Windows in coming releases.
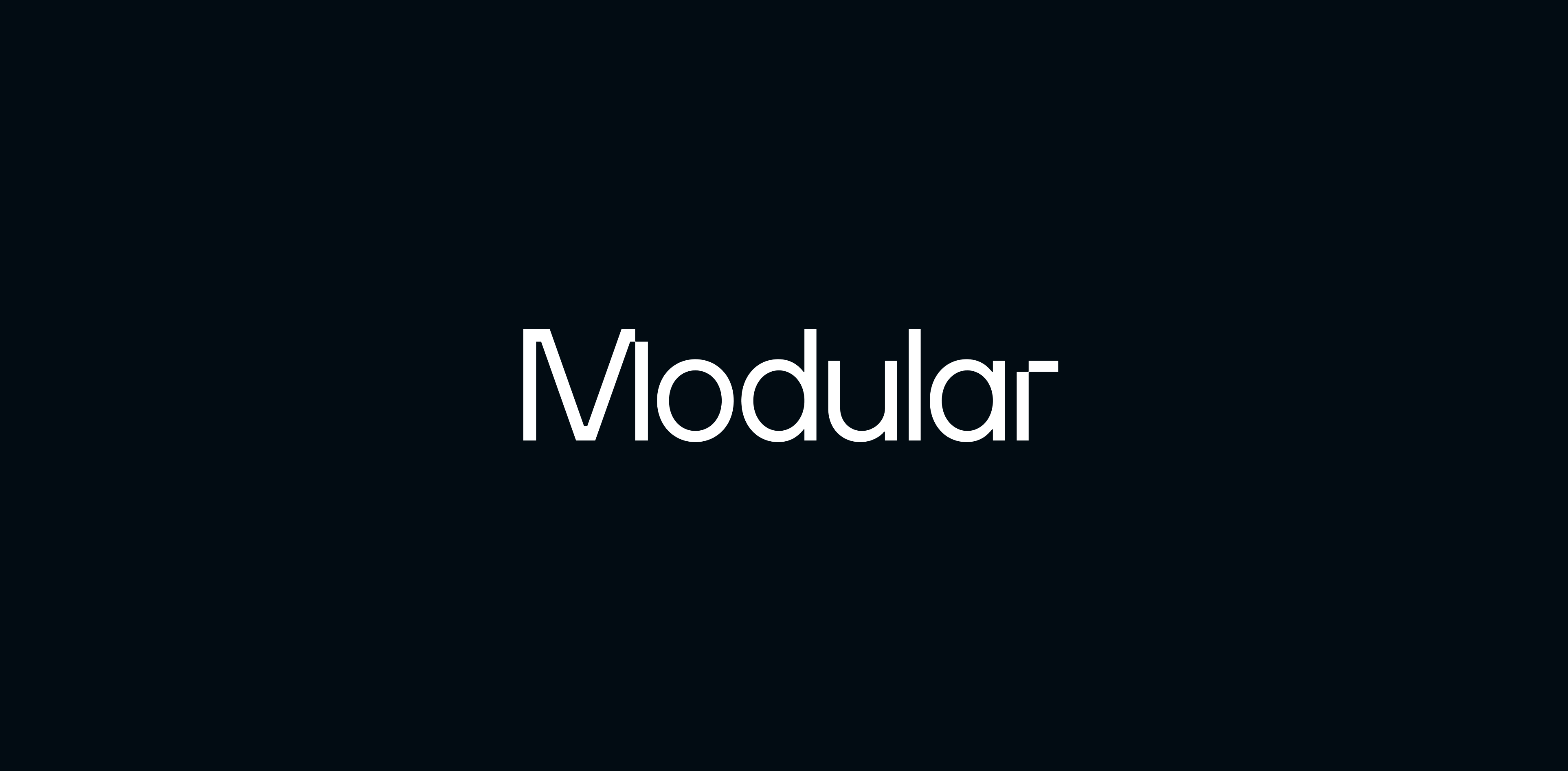
We’ve raised $100M to fix AI infrastructure for the world's developers
We are excited to announce that we have raised $100 million in new funding, led by General Catalyst and filled by existing investors GV (Google Ventures), SV Angel, Greylock, and Factory. This second round of funding follows our first $30 million round from last year and will enable us to supercharge our vision for the future of AI infrastructure for the world's developers.

An easy introduction to Mojo🔥 for Python programmers
Learning a new programming language is hard. You have to learn new syntax, keywords, and best practices, all of which can be frustrating when you’re just starting. In this blog post, I want to share a gentle introduction to Mojo from a Python programmer’s perspective.
.jpg)
What’s the difference between the AI Engine and Mojo?
On May 2nd, we announced our next-generation AI developer platform with two exciting breakthrough technologies — the Mojo programming language and the Modular AI Engine. In just over two months, more than 110k developers have signed up for the Mojo Playground to learn Mojo and experience its performance firsthand, over 30k developers have signed up to our waitlist for the AI engine, and our Modular community on Discord has grown to 17k developers! We’re incredibly excited to see developers sharing their experience with Mojo, providing product feedback, and learning from each other.
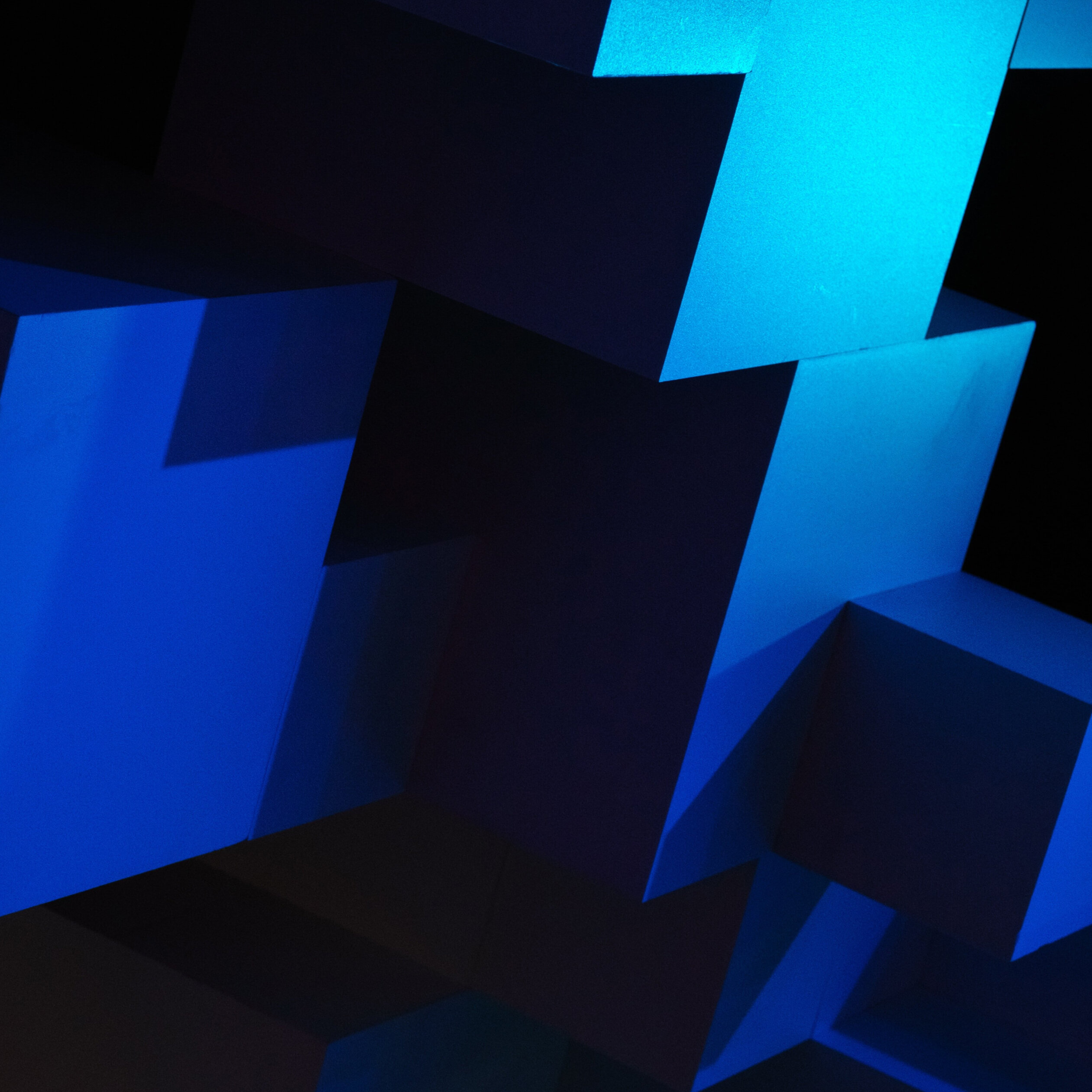
Modular natively supports dynamic shapes for AI workloads
Today’s AI infrastructure is difficult to evaluate - so many converge on simple and quantifiable metrics like QPS, Latency and Throughput. This is one reason why today’s AI industry is rife with bespoke tools that provide high performance on benchmarks but have significant usability challenges in real-world AI deployment scenarios.
Start building with Modular
Quick start resources
Get started guide
With just a few commands, you can install MAX as a conda package and deploy a GenAI model on a local endpoint.
Browse open source models
500+ supported models, most of which have been optimized for lightning fast speed on the Modular platform.
Find examples
Follow step by step recipes to build Agents, chatbots, and more with MAX.