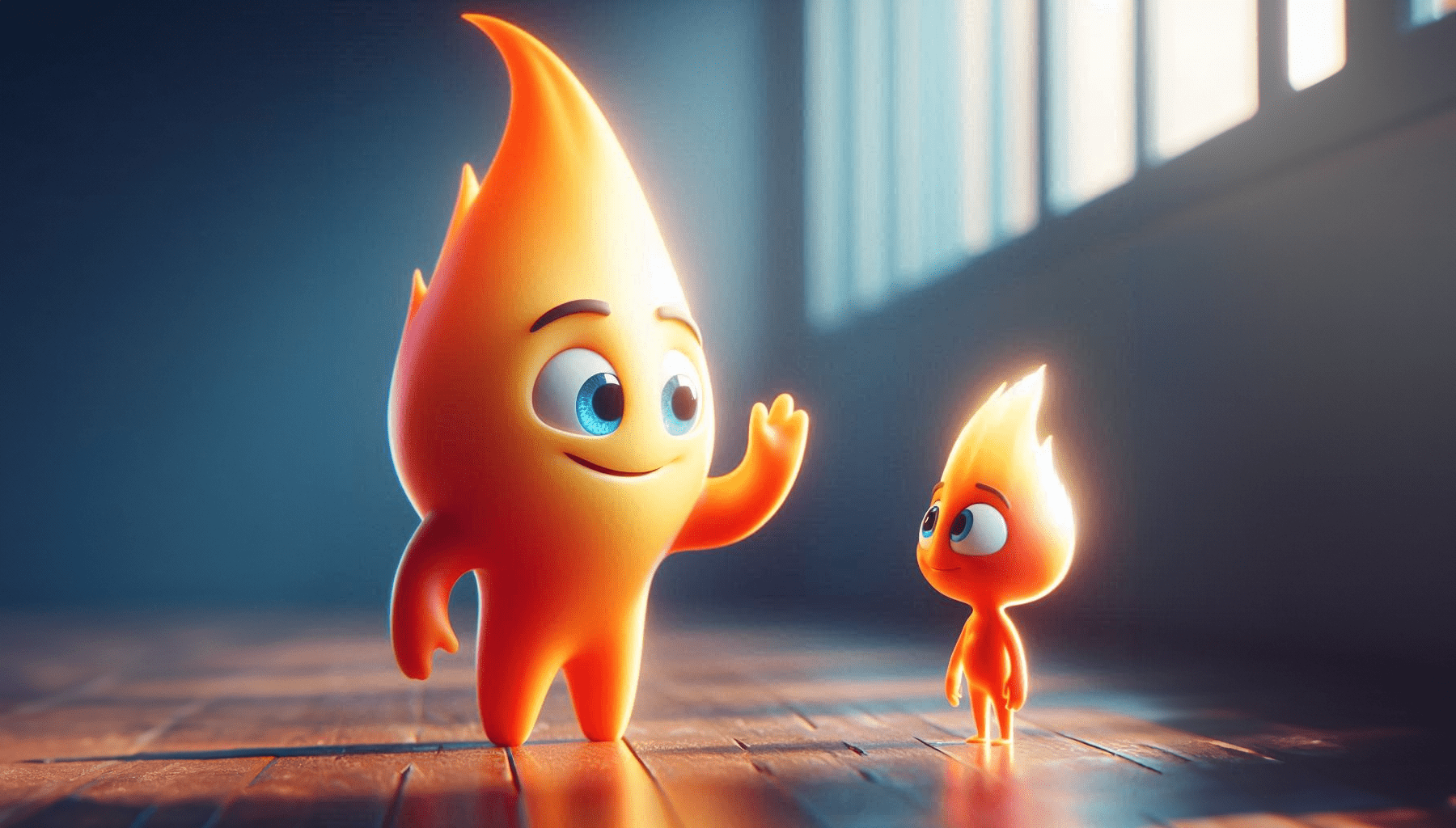
What’s new in Mojo 24.4? Improved collections, new traits, os module features and core language enhancements
Mojo 24.4 is now available for download, and this release includes several core language and standard library enhancements. In this blog post, we’ll dive deep into many of these features using code examples. One of the biggest highlights of this release is that we received 214 pull requests from 18 community contributors for new product features, bug fixes, documentation enhancements, and code refactoring. These contributions resulted in 30 net new features in the standard library, accounting for 11% of all improvements in this release. We’re incredibly proud of the momentum we’re seeing with community contributions, and it goes without saying – you are the real star of this release. On behalf of the entire Mojo team, we’d like to thank you for all your contributions to making Mojo awesome!

Deep dive into ownership in Mojo
This post blog is the second part of the series of ownership in Mojo. Please make sure to check out the first part, What Ownership is Really About: A Mental Model Approach, as we will build on concepts developed there. This post serves as accompanying material for the deep dive on ownership by our CEO, Chris Lattner. Be sure to watch the video as well, which covers how ownership is implemented in Mojo's compiler, providing further insights and technical details.

What ownership is really about: a mental model approach
Ownership is a well-known concept in modern programming languages such as Mojo that aims to provide a safe programming model for memory management while ensuring high performance. This allows programmers to build safe abstractions without the need to manually manage memory, making development more efficient and less error-prone.
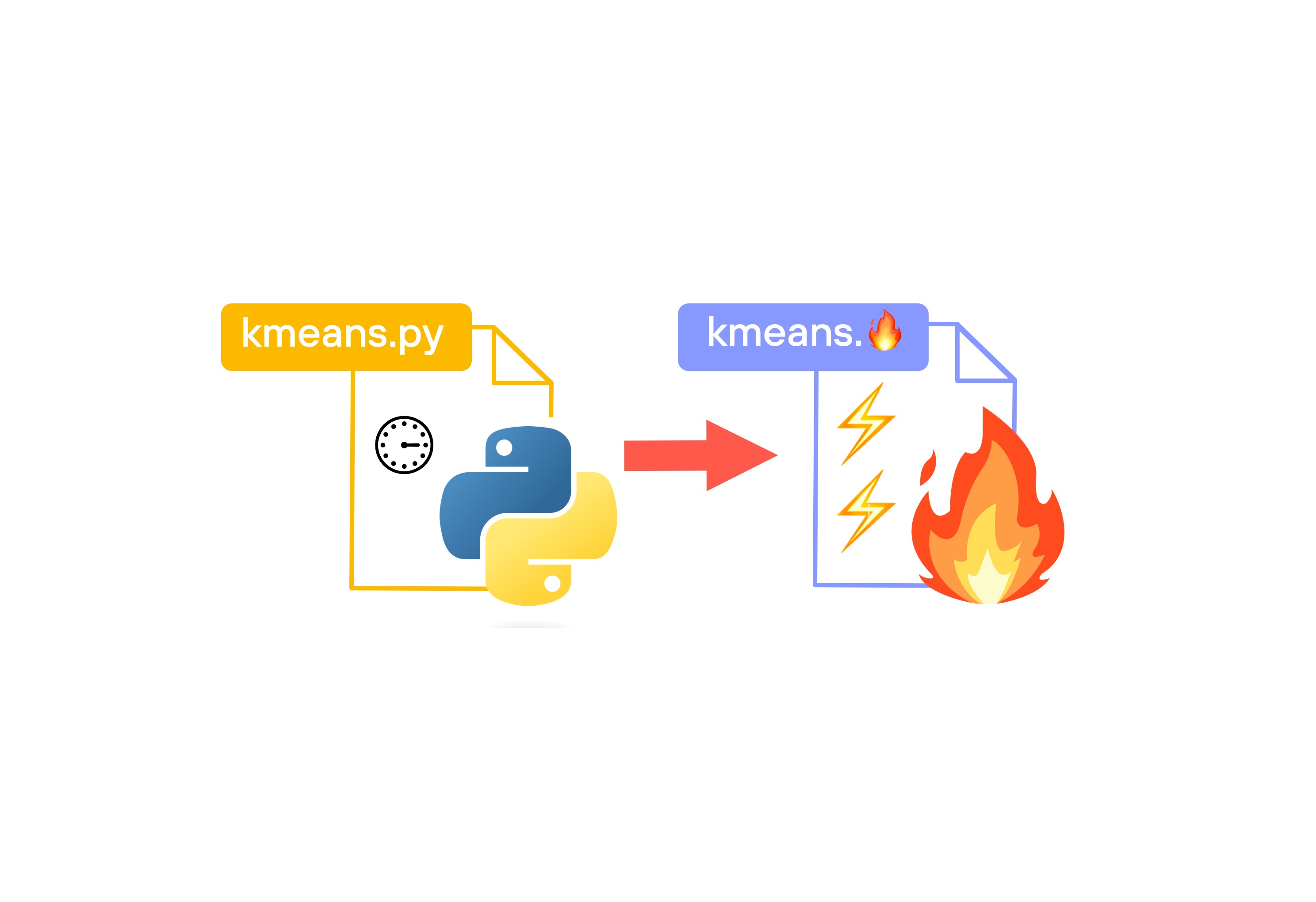
Fast⚡k-means clustering in Mojo🔥: a guide to porting Python to Mojo🔥 for accelerated k-means clustering
There are several clustering algorithms, but k-means — the algorithm we're going to implement from scratch in Python and Mojo🔥 in this blog post — is one of the most popular due to its simplicity and ease of implementation.

What’s New in Mojo 24.3: Community Contributions, Pythonic Collections and Core Language Enhancements
Mojo🔥 24.3 is now available for download and this is a very special release. This is the first major release since Mojo🔥 standard library was open sourced and it is packed with the wholesome goodness of community contributions! The enthusiasm from the Mojo community to enhance the standard library has been truly remarkable. And on behalf of the entire Mojo team, we’d like to thank you for all your feedback, discussion and, contributions to Mojo, helping shape it into a stronger and more inclusive platform for all.
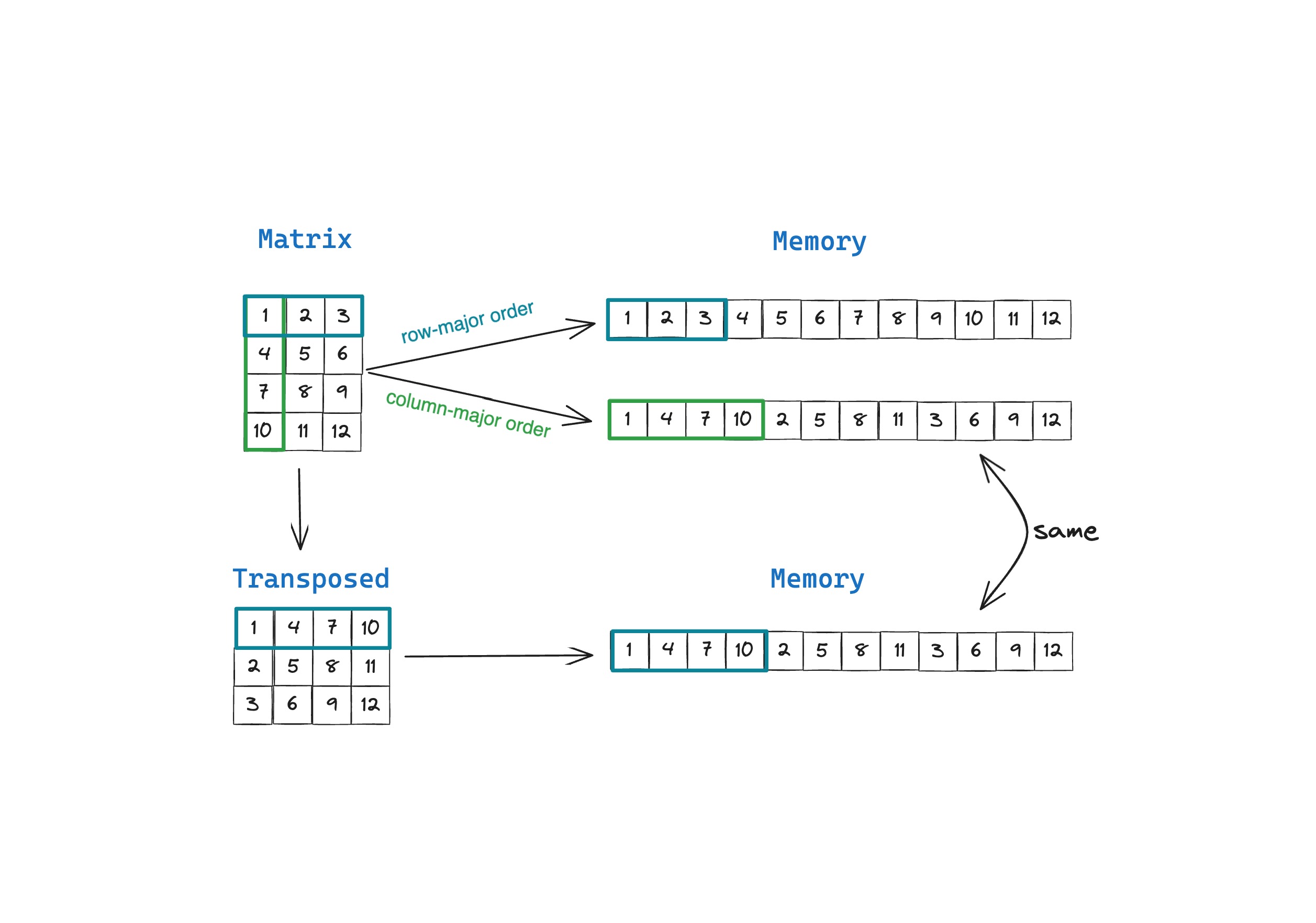
Row-major vs. Column-major Matrices: A Performance Analysis in Mojo and NumPy
A matrix is a rectangular collection of row vectors and column vectors that defines linear transformation. A matrix however, is not implemented as a rectangular grid of numbers in computer memory, we store them as a large array of elements in contiguous memory.
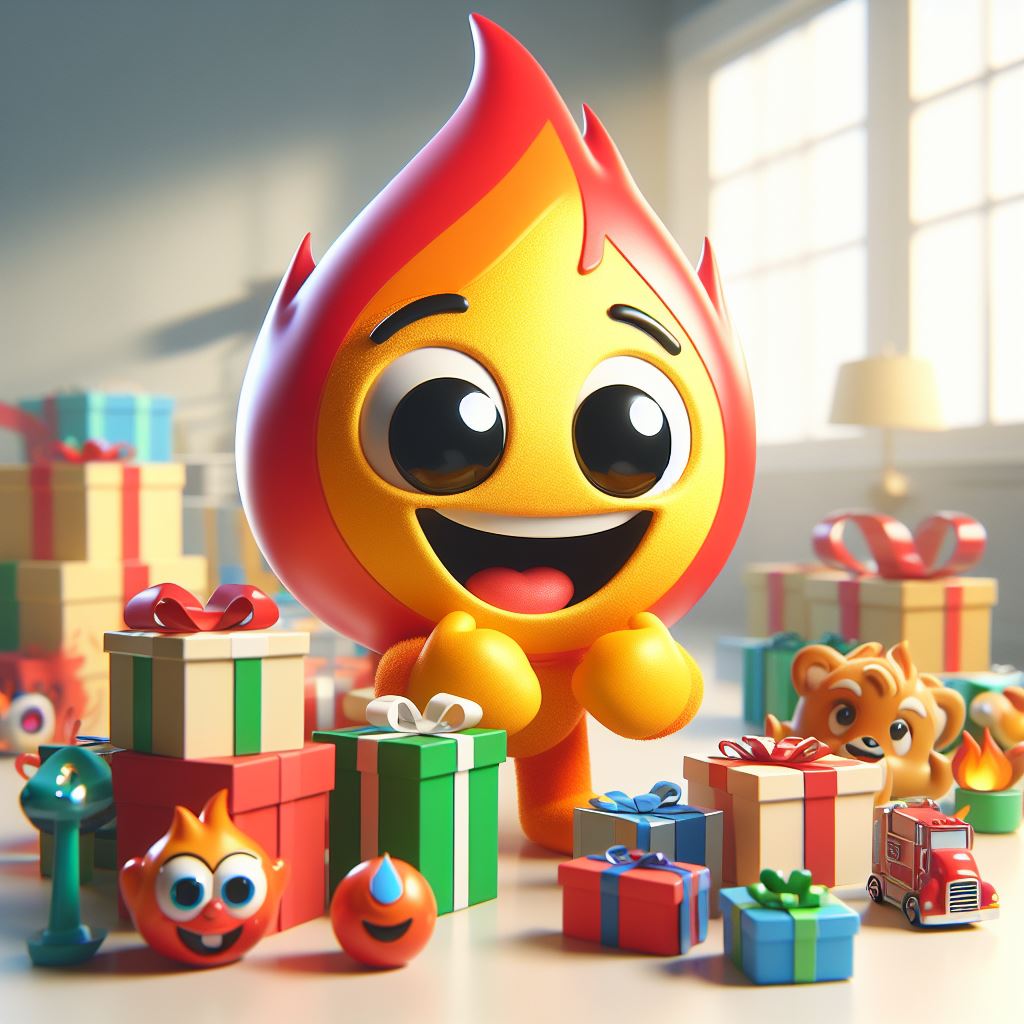
What’s new in Mojo 24.2: Mojo Nightly, Enhanced Python Interop, OSS stdlib and more
This will be your example-driven guide to Mojo SDK 24.2, as part of the latest MAX release. If I had to pick a name for this release, I’d call it MAXimum⚡ Mojo🔥 Momentum 🚀 because there is so much much good stuff in this release, particularly for Python developers, adopting Mojo.
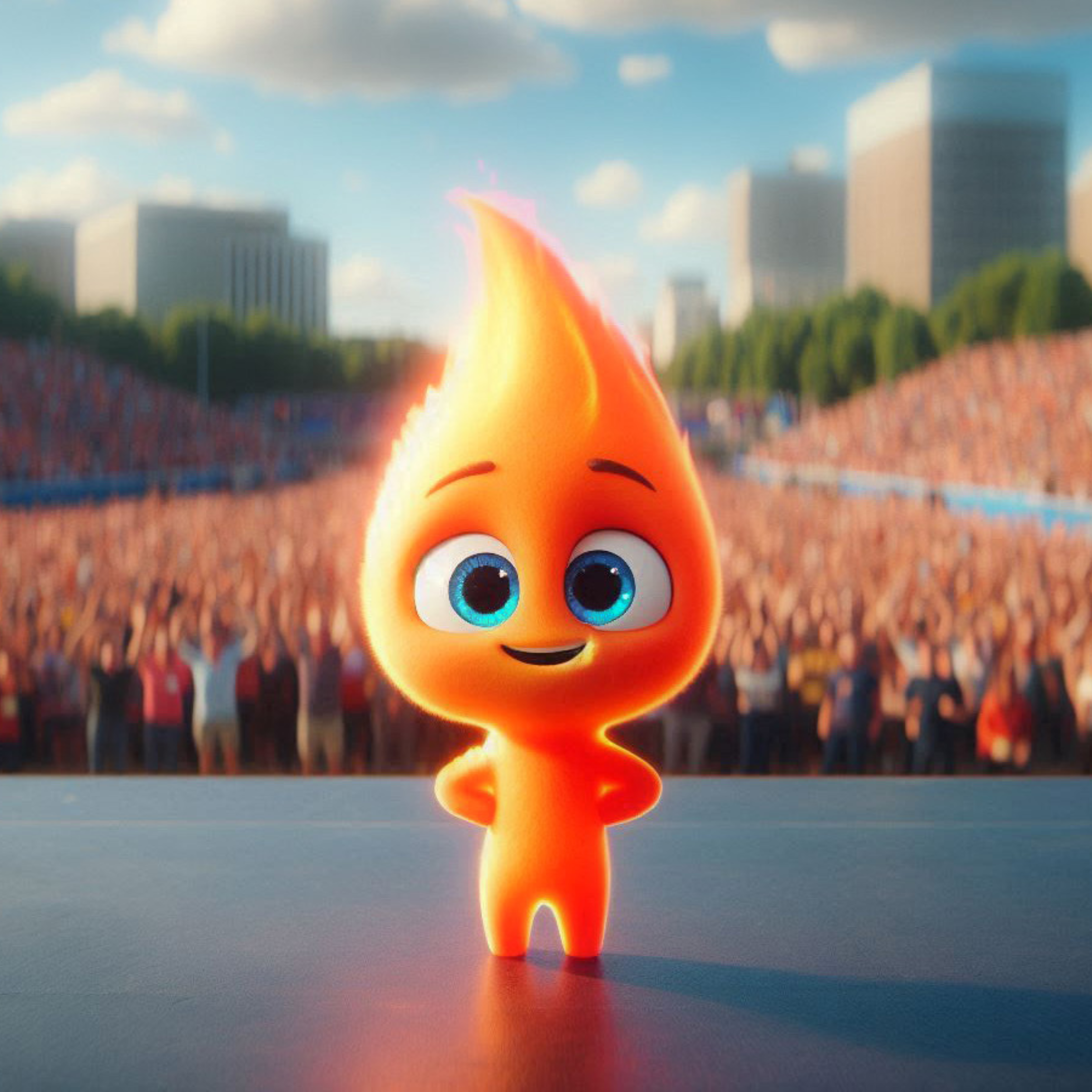
The Next Big Step in Mojo🔥 Open Source
At Modular, open source is ingrained in our DNA. We firmly believe for Mojo to reach its full potential, it must be open source. We have been progressively open-sourcing more of Mojo and parts of the MAX platform, and today we’re thrilled to announce the release of the core modules from the Mojo standard library under the Apache 2 license!

Semantic Search with MAX Engine
In the field of natural language processing (NLP), semantic search focuses on understanding the context and intent behind queries, going beyond mere keyword matching to provide more relevant and contextually appropriate results. This approach relies on advanced embedding models to convert text into high-dimensional vectors, capturing the complex semantics of language.
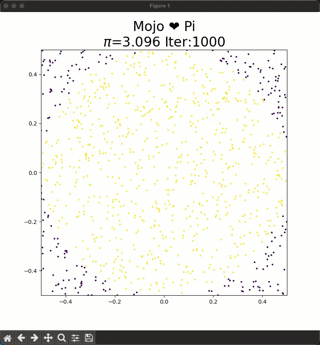
Mojo🔥 ❤️ Pi 🥧: Approximating Pi with Mojo🔥 using Monte Carlo methods
March 14th aka 3/14 or 3.14 is known as $\pi$ Day, and it honors the mathematical constant $\pi$ (pi), which represents the ratio of a circle's circumference to its diameter. On this special day, I wanted to dedicate a blog post to the beauty of mathematics, numerical methods, $\pi$, and Mojo. So join me on this journey as I implement a fast vectorized Monte Carlo approximation method of calculating $\pi$. Happy $\pi$ Day!
Easy ways to get started
Get started guide
With just a few commands, you can install MAX as a conda package and deploy a GenAI model on a local endpoint.
400+ open source models
Follow step by step recipes to build Agents, chatbots, and more with MAX.
Browse Examples
Follow step by step recipes to build Agents, chatbots, and more with MAX.